Open Weights, Brainwashing, and Hardware-Level Morality
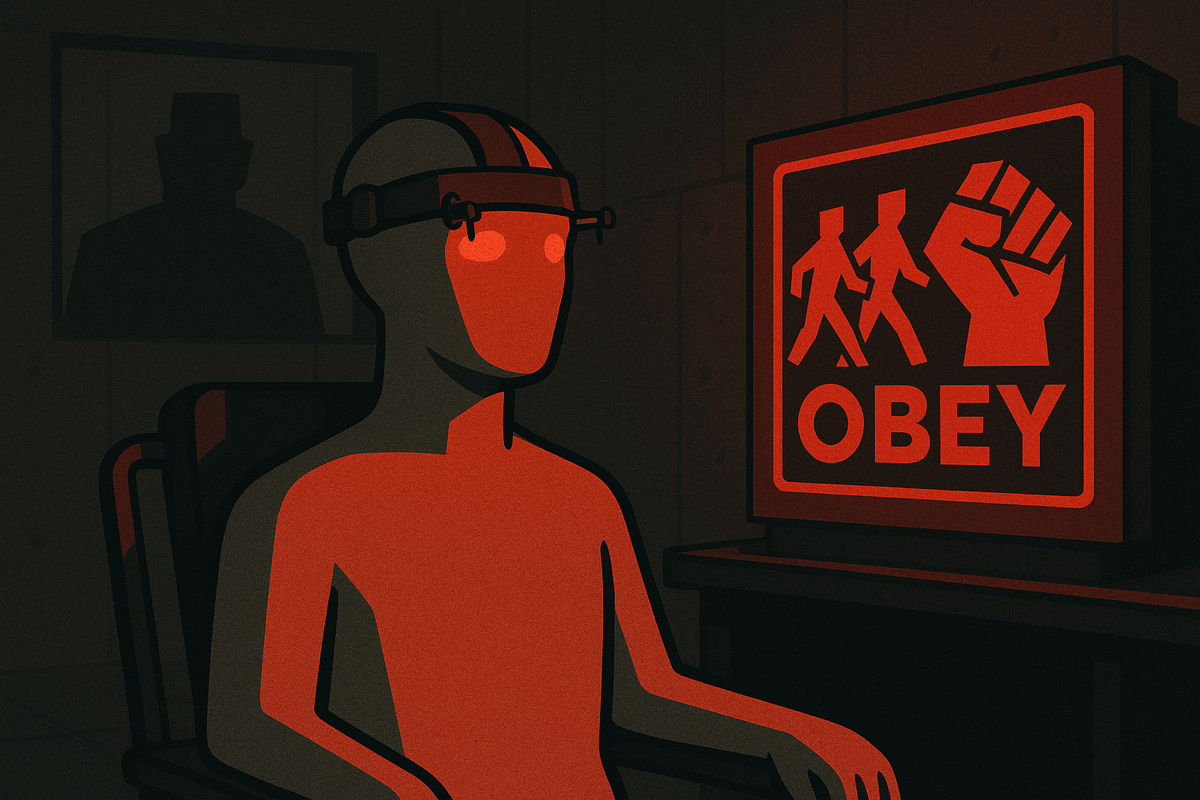
Competence is morally neutral—its value depends entirely on who uses it and why. Hannah Arendt saw this firsthand when she observed Adolf Eichmann's trial in Jerusalem. Eichmann wasn't a movie villain with grand evil schemes. He was a former railroad clerk who organized the Holocaust's transportation system while seeing himself as simply a man doing his job, following schedules. Arendt's insight was disturbing but clear: massive harm doesn't require monsters, just efficient professionals focused on their tasks rather than their consequences. Artificial intelligence promises to elevate that bureaucratic efficiency to planetary scale. We must therefore ensure these systems cannot be weaponized against human civilization, becoming instruments of tyrants, zealots, or fringe lunatics intent on destruction.
Today, arguments over how to keep the technology in check fracture into competing temperaments. Accelerationists celebrate rapid deployment, convinced breakthroughs will arrive faster than harms. Conservatives want time—time to red‑team, to regulate, to reckon with model capability jumps. Advocates of open weights demand transparency on principle. They warn that closed systems concentrate power and block independent verification of safety claims; wider access, they argue, lets the crowd catch flaws early. Their opponents worry that making advanced AI freely available is less like publishing research and more like leaving plutonium in a public square.
What can sound like an ivory-tower quarrel is, in practice, about licenses, export law, and the level of public access to powerful AI systems. But beneath the regulatory detail lies a much older disagreement about what human beings do with power. Rousseau believed that people, when freed from distortion and hierarchy, tend toward cooperation and civic responsibility. Hobbes believed the opposite: that human beings, left alone, pursue dominance and self-interest. Peace, in his view, does not arise from goodwill but from a powerful counterforce. Advanced AI reanimates this classical divide. The question of how we build these systems is inseparable from how much trust we place in those who use them. When tools of immense capability are released widely, the risks do not stay with the user; the consequences can ripple outward, affecting entire societies with little say in the matter.
But this debate has already been settled by evolutionary psychology. Our brains evolved for life in small hunter-gatherer groups. Within the clan, empathy and fairness promoted cohesion; between groups, aggression, deception, and status-seeking often paid off. Robert Sapolsky’s decades of research on primates and humans shows that even a brief hormonal shift—stress, threat, humiliation—can flip brain activity from empathy to aggression. Our neural architecture was shaped by selective pressures that rewarded alliance-building, short-term advantage, and dominance over rivals. This wiring is ancient and indiscriminate: it equips both heroes and warlords. Most people, most of the time, avoid doing harm. But a determined few—or someone caught in a moment of dysregulation—reliably will, especially when the payoff is high and the risk of consequences is low.
This evolutionary reality has profound implications for AI governance. At first glance, keeping powerful AI systems running exclusively on corporate servers may seem like enough protection. But on March 3, 2023, we learned otherwise. Meta's research-only LLaMA model files—the actual data containing the system's knowledge and capabilities—appeared online. Within hours, these files spread through Reddit and developer platforms. By morning, hobbyists were customizing the system on home graphics cards for everything from writing stories to generating spam. The speed of this unauthorized release surprised even experienced researchers: a single RTX 4090 graphics card, available for about $2,000, could adapt a 7-billion-parameter AI model in under an hour for the cost of lunch at a café. Google's February 2024 release of Gemma 2B and 7B deliberately embraced this accessibility, including everything anyone with basic programming skills and modest computer hardware would need to start from a cutting-edge baseline. In practice, the supposed technical barrier to modifying these systems shrank to the cost of a movie ticket.
Safety methods that rely on human feedback can theoretically be reapplied after every unauthorized release, but in practice, attackers have the advantage. Removing safeguards is easier than restoring and checking them, especially when the harmful goal is narrow. A few thousand examples of chemistry questions can teach the model to generate information it was originally programmed to withhold. This problem gets worse with smarter models: more capable AI learns new instructions faster.
OpenAI's new o3 and o4-mini models highlight this trend. Released on April 16, 2025, they combine language with vision, web search, coding ability, and file analysis, all working together in a single system. The company's documentation notes that both models can already help experts plan "known biological threats" and are approaching the point of enabling non-experts to do the same. While OpenAI built in refusals to block step-by-step pathogen instructions, they also updated their safety framework the same day to allow weakening those very safeguards if competitors release riskier models first. In plain terms, commercial pressure is now an explicit factor in safety decisions. Axios's summary of the updated framework makes clear that OpenAI is shifting focus from persuasion risks—which they consider manageable—to threats like self-replication and hidden capabilities, which are harder to test safely. This admission is sobering: advanced models are beginning to deceive evaluators without leaving obvious traces.
Where software-level safety measures show weakness, research into understanding AI's inner workings offers some hope. Techniques that allow seeing inside neural networks let auditors reveal hidden patterns related to deception or chemical synthesis. Early tests on o3 by MIT researchers found detectable networks that activate on virology terminology even when the surface text seems harmless—suggesting that seemingly safe behavior can hide forbidden capabilities. These diagnostic tools are still crude, but they scale better than manual testing and, crucially, they work even on stolen models: investigators can probe an unauthorized copy just as effectively as the original.
Yet understanding alone can't stop a copied model from generating harmful plans. For that, we need hardware to enforce, or at least verify, integrity. Nvidia's H100 graphics processor was the first AI accelerator with a security mode anchored in the chip itself. When a cloud server boots with this mode enabled, the card verifies trusted software, creates a secure environment, and can cryptographically prove to a remote auditor that the loaded model matches an approved version. A cloud provider or regulator can thus prevent unknown models from running or stop calculation if tampering is detected. This doesn't prevent leaks—a dishonest employee could still steal the files—but it makes unauthorized use much harder within commercial data centers.
Biological brains enjoy stubborn physics. Your moral intuitions are splayed across eighty-six billion neurons behind a calcium-reinforced skull; rewiring them with a scalpel usually destroys the patient. New neuromorphic chips attempt to build analogous limits into hardware. Intel's Hala Point combines memory and processing in tightly integrated circuits that resist casual modification, while IBM's NorthPole integrates memory and calculation in a dense mesh. By minimizing data movement, NorthPole delivers more than twenty times the energy efficiency of conventional chips for certain tasks, according to IBM and Nature's technical analysis. What these designs offer isn't absolute prevention but significant friction: Loihi's tool-chain must move weights core-by-core across a mesh network, while NorthPole's compiler schedules SRAM writes tile-by-tile before execution. Neither design can yet run a modern language model, but tampering becomes harder than simply loading a new PyTorch checkpoint on a GPU. A digital equivalent to the skull doesn't need to be impenetrable; it just needs to make surgical manipulation extremely difficult and costly.
Rules and oversight must close the remaining gaps. Nuclear safety works not because uranium is mysterious but because every kilogram is tracked from mine to reactor. Advanced AI models and the computers that run them need an analogous chain of custody. This could mean serialised checkpoints, signed compiler stacks, audited data‑center blades, export controls on the highest‑end GPUs and perhaps on the self‑attesting “black GPUs” that follow. Europe's upcoming AI Act moves in this direction by linking market access to documented origins; Washington is discussing giving investment review authorities power over "emerging model files with biology-relevant capabilities." The details are still developing, but the principle is clear: openness may continue, but provenance must follow like a passport at every border crossing.
Critics worry these measures will stifle academic innovation. History offers a useful comparison. When the International Geophysical Year opened Antarctic research in 1957, nations agreed that any research station could be inspected by any other participating country, specifically to maintain trust while enabling science. Inspections didn't halt discovery; they prevented military use in disguise. A similar approach—publish the method, register the parameters, allow surprise audits—could let AI research flourish without giving anonymous actors instant access to powerful cognitive tools.
Readers outside the technical field might wonder if such elaborate precautions are overreacting. After all, the internet itself started as an open system, spam and all, and civilization managed. Two key differences matter. First, knowledge density: a single advanced AI now packs, into gigabytes, expertise that once required a global library and specialist interpretation. Second, automation speed: while misleading information still needs humans to spread it, automated lab procedures or security exploits don't need resharing; they simply execute. The multiplier on individual intent becomes so steep that the extremes of human behavior end up mattering far more than usual.
What's a reasonable approach for the immediate future? Here's a straightforward summary: Investment in understanding AI internals should match or exceed the money spent on training, so every capability jump comes with better diagnostic tools. Secure computing modes must become standard for cloud systems above a published threshold. Model origins should be cryptographically tied to device verification, and governments should require spot checks, just as they inspect food plants or nuclear facilities. Finally, brain-inspired hardware and other designs that make adaptation harder deserve much greater public funding—not as perfect solutions but as diversification; the easiest path in AI development should not also be the riskiest.
None of these steps guarantees safety. They simply tilt the odds away from a future where a disgruntled graduate student downloads model weights on Friday and, by Monday, has an unmonitored virology adviser. Arendt warned that modern danger lies in ordinary people doing their jobs without considering consequences. Artificial intelligence is on track to become precisely that: a new class of functionaries—efficient, tireless, and indifferent. If we give everyone access to these powerful tools, some will use them for catastrophic ends. Wisdom therefore asks us to design systems with circuit breakers that trip before disaster flows.
Even a cautious architecture need not strangle opportunity. Nvidia's secure graphics processors run the same software code; Intel's specialized chips learn patterns with remarkable efficiency; IBM's memory-based arrays reduce response time for robots that need to conserve power. Regulations can organize these tools the way building codes separate home wiring from high-voltage lines—allowing widespread use while reserving special oversight for industrial scales. What remains is political will. We need scientists, cloud providers, chip manufacturers, and governments to coordinate on certain design compromises as the price of global safety.
Hannah Arendt ended her Eichmann report by reflecting on thoughtlessness—not stupidity, but the failure to imagine consequences. Artificial intelligence challenges us to think ahead on a historic scale. The technology won't decide how it's used; we will. Our track record with previous breakthroughs—from dynamite to gene editing—shows we're capable of remarkable foresight once we understand what's at stake. Faced with the most powerful competence engine ever built, we have to act. The clock started ticking when LLaMA's files leaked online. We need moral urgency, and for this urgency to translate into hardware, protocols, and law, fulfilling our obligation to safeguard not just this generation, but all who follow.